Shopify Customer Cohorts: A Comprehensive Guide
Customer Cohort Analysis became an essential tool for successful direct-to-consumer brands on Shopify to monitor the customer behavior and improve customer retention.
At a time when customer acquisition is becoming increasingly expensive, it's even more important to focus on retaining existing customers. By focusing on customer retention, your brand can reduce the cost of customer acquisition and increase sales through repeat purchases and upselling. In addition, customer retention can improve your understanding of customer needs.
RetentionX helps your brand track and understand customer behavior patterns, make informed decisions, and identify valuable group of customers for retention and acquisition.
-
RetentionXMaximize Your Lifetime Value (LTV) with Analytics & Automation
30-day free trial
Free plan available
Built for Shopify Plus
For example, let's say a brand wants to analyze the behavior of customers who made their first purchase in 2020. They would group customers that bought in their Shopify store into cohorts based on the month they made their first purchase. The cohort analysis table might look something like this:
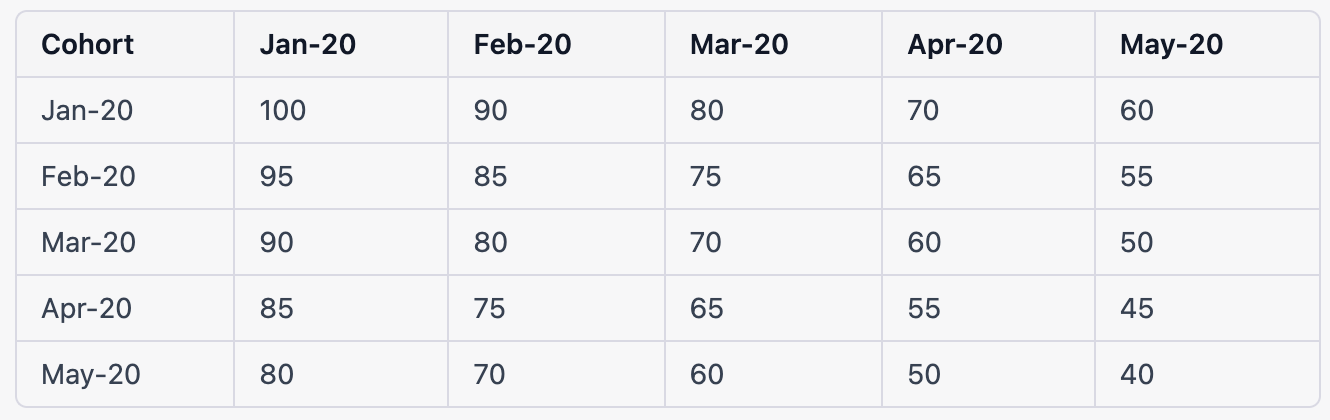
In this monthly cohort analysis report, the first column represents the cohort (the month the customer made their first purchase), and the following columns represent the percentage of customers who made a repeat purchase in your online store in the following months.
We can see that the cohort of customers who made their first purchase in January have a higher percentage of repeat purchase as time goes by. On the other hand, the cohort of customers who made their first purchase in May have a lower percentage of repeat purchase as time goes by.
By analyzing this table, the brand can see that customers who make their first purchase in January are more likely to make repeat purchases, while customers who make their first purchase in May are less likely to do so.
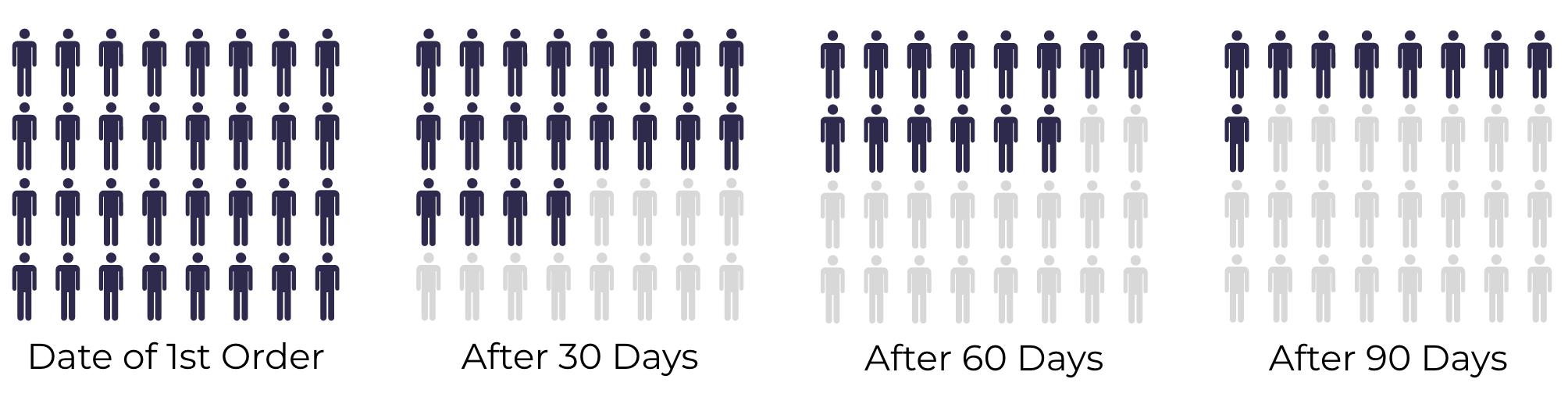
Overall, early detection of discrepancies in customer behavior is important for your brand because it allows you to be proactive in addressing potential issues, rather than reactive. By identifying and addressing potential issues early on, your brand can prevent churn, reduce the number of one time buyers and improve your overall customer retention rate.
For example:
- if you notices that a particular customer cohort is beginning to make fewer purchases or has a higher number of customer service interactions, you can take action to address the underlying issues before the customers decide to stop doing business your brand.
- if your brand notices that a particular customer cohort is increasingly interested in a new product category, you can take steps to capitalize on that trend by developing new products or features to meet that demand.
– Shopify customer cohorts don't provide all the filters and granularity you need out of the box.
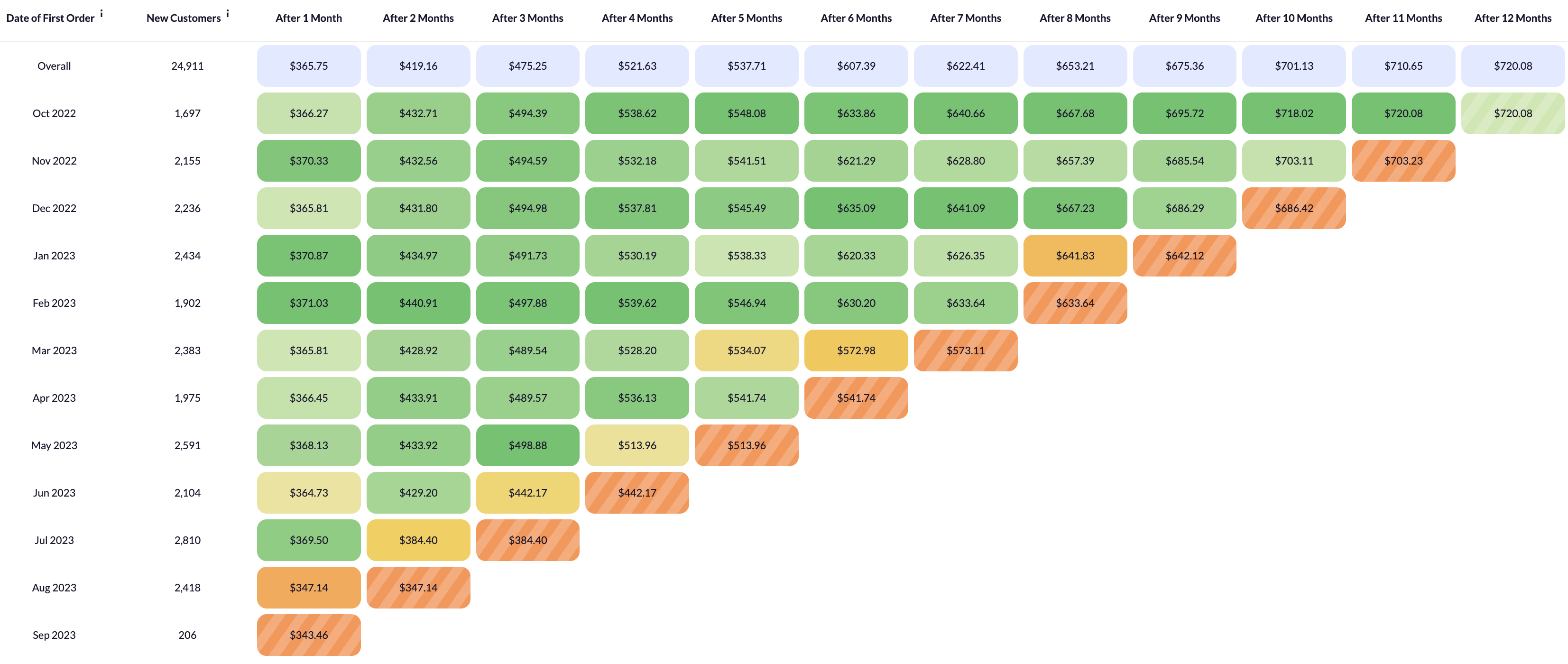
It's worth noting that the process of creating a cohort analysis may vary depending on the tool or software you are using. The most important thing is to have a clear understanding of what you want to achieve and how the customer data can help you achieve it.
Step1: Define the cohorts
The first step is to define each group of customers that you want to analyze. This can be based on a variety of factors, such as purchase date, product category or demographic data.This can be easily done in RetentionX using the integrated customer segment tool.

Examples for interesting customer segments to analyze would be:
- Time cohorts: Analyze how your customer behavior has changed over time and whether your measures to improve customer retention are successful. To do this, group customers by the month of their first purchase.
- Product cohorts: Customers who buy certain products on their first purchase (SKU of first purchase). This metric is often also referred to as product LTV, as it indicates the quality of customers who buy a particular product on their first purchase.
- Coupon cohorts: Customers redeeming certain coupons with their purchase.
- Subscription cohorts: for subscription commerce brands – subscribers vs. non-subscribers
- Demographic cohorts: segmentation of customers by city, age and other demographic characteristics, often generated via customer surveys.
- Marketing cohorts: Customers acquired through specific marketing channels (influencers, social ads, …)
Adding 'predicted spend tier' as a criterion for segmenting customers can significantly enhance targeting efforts by identifying high-value customers for more focused marketing strategies.
Step 2: Building a cohort analysis report
You can look at cohorts and identify your most loyal customers according to different criteria depending on your goal:
Cohorts by Average
Cohorts on average show how an average customer from a cohort behaved after a certain period of time (e.g. customer lifetime value per customer).
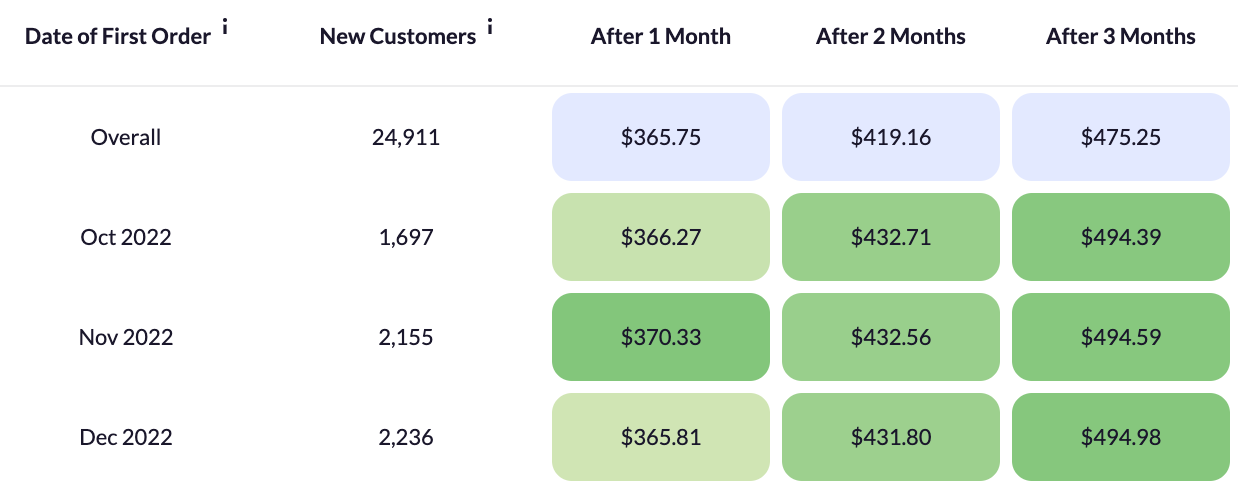
This example is about the average Customer Lifetime Value of a customer acquired in a specific month. Customers acquired in February 2022 have a higher lifetime value (net sales excl. taxes - discounts - shipping - cost of goods) than customers acquired in March or April. Looking at cohorts in this way gives you a good indicator of how the average quality of your customer base is evolving over time.
Cohorts by Total
Cohort analysis by total shows you how much gross sales & net sales were generated by a particular customer group.
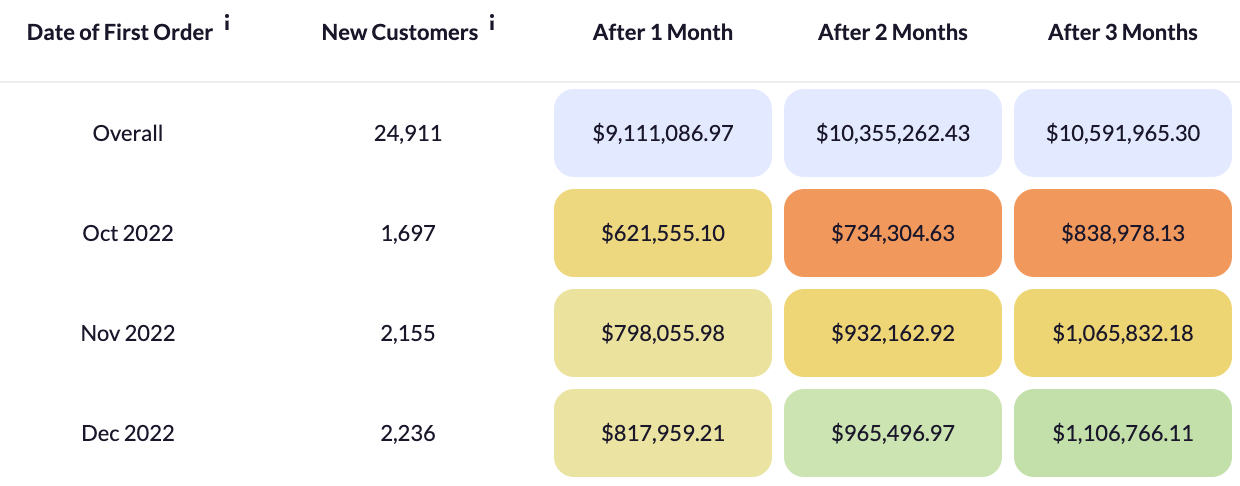
Monthly Cohort by Retention Rate
Customer cohorts by retention rate show how many of the customers acquired in a given month placed a second order within a given time period.
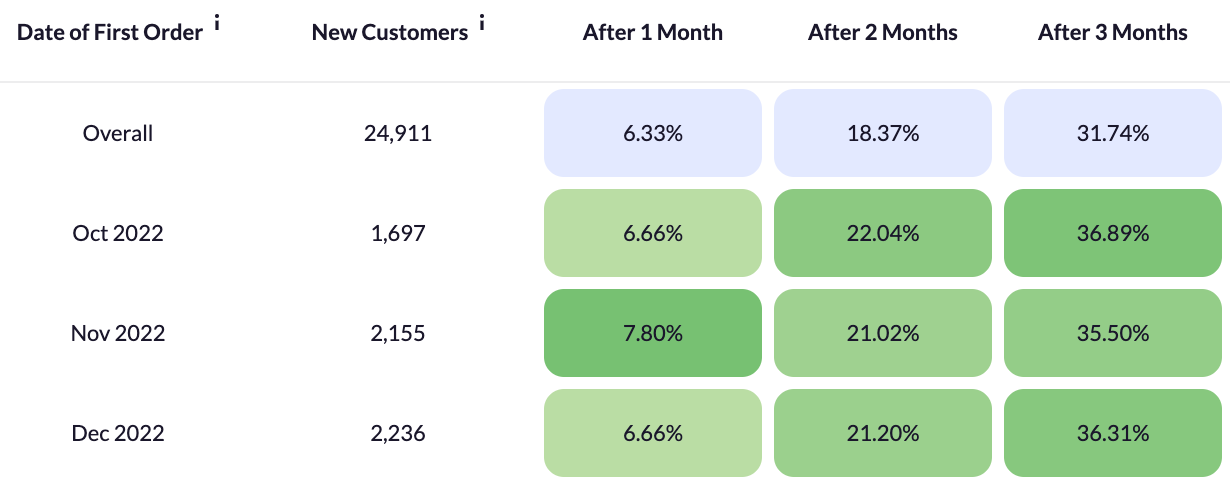
In this example, 21.82% of February 2022 customers placed a second order within 2 months. This analysis is particularly important to understand how quickly a second order is completed and how likely a particular customer group is to complete the next order based on the performance of previous cohorts.
Cohorts by Repeat Purchase Rate
Cohort by repurchase rate shows the percentage of customers in a given group who complete a subsequent order.
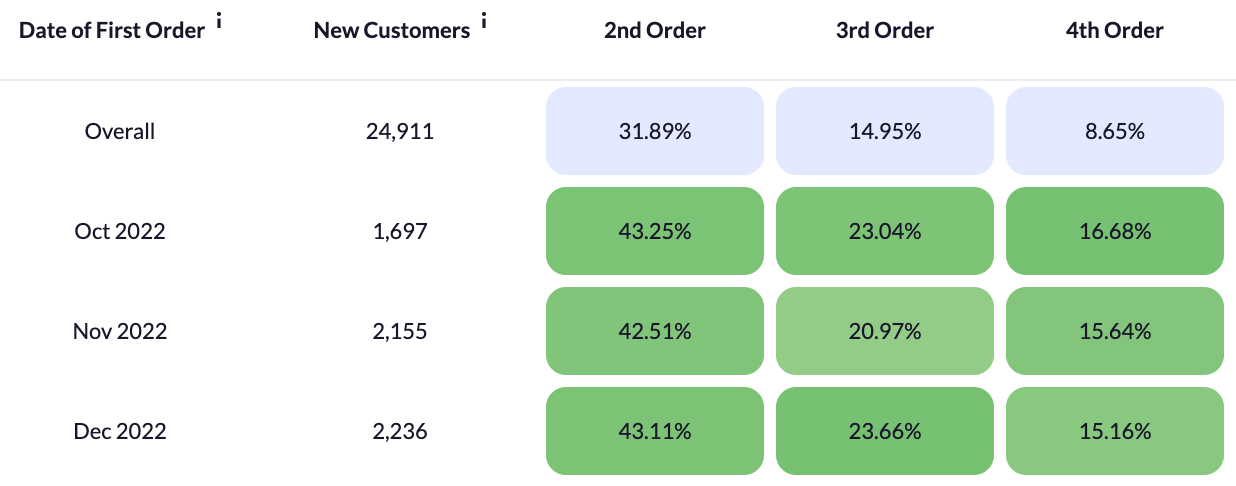
In February 2022, 44.43% of customers have placed a second order. 18.37% have already placed a fourth order. This analysis shows the performance of the cohort for each subsequent order, regardless of the time between orders.
Other potential cohort analysis tables may include:
- AOV (Average Order Value) by Cohort
- Cohorts by Sales Channels
- Product Return Rate by Cohort
-
RetentionXMaximize Your Lifetime Value (LTV) with Analytics & Automation
30-day free trial
Free plan available
Built for Shopify Plus
Step 3: How to recognize early warning signs of churn
- Decrease in purchase frequency: A decrease in the number of purchases made by a customer over time can indicate that they are losing interest in your products or services.
- Low lifetime value: A low lifetime value, calculated as the total revenue generated by a customer over their lifetime, can indicate that they are not as engaged with your brand as other customers.
- High customer service interactions: A high number of customer service interactions can indicate that a customer is experiencing problems or dissatisfaction with your products or services.
- Decrease in purchase value: A decrease in the average purchase value made by a customer over time can indicate that they are becoming less engaged with your brand or that they are experiencing financial difficulties.
- Inactivity: Customers who have not made a purchase or interacted with your brand in a long time are at risk of churning.
Step 4: Take action

Based on the results of the analysis, you can take action to improve the customer experience and customer lifetime value, such as creating targeted marketing campaigns, developing new products, or optimizing pricing and promotions. For this, create a segment of customers you want to reactivate and synchronize it to audiences in your favorite marketing & CRM tools:

- Reengage customers with targeted a marketing strategy: Reach out to customers who have exhibited early signs of churn risk and offer them incentives to continue doing business with your brand.
- Address customer issues: Address any issues that may be causing dissatisfaction, such as poor customer service or product defects.
- Personalize the customer experience: Personalize the customer experience by providing tailored recommendations and offers to retain customers longer and increase customer lifetime value.
- Monitor and track customer behavior over time: Continuously monitor and track customer behavior over time to identify any changes in behavior that may indicate an increased risk of churn.
Shopify's internal cohort reporting can be found under Analytics > Reports > Customer Cohort Analysis. While Shopify also offers cohort analysis itself, it is unfortunately very limited. Only simple filter conditions are possible for customer segmentation, which cannot be linked with each other. There is also no way to take action on these cohorts and findings.
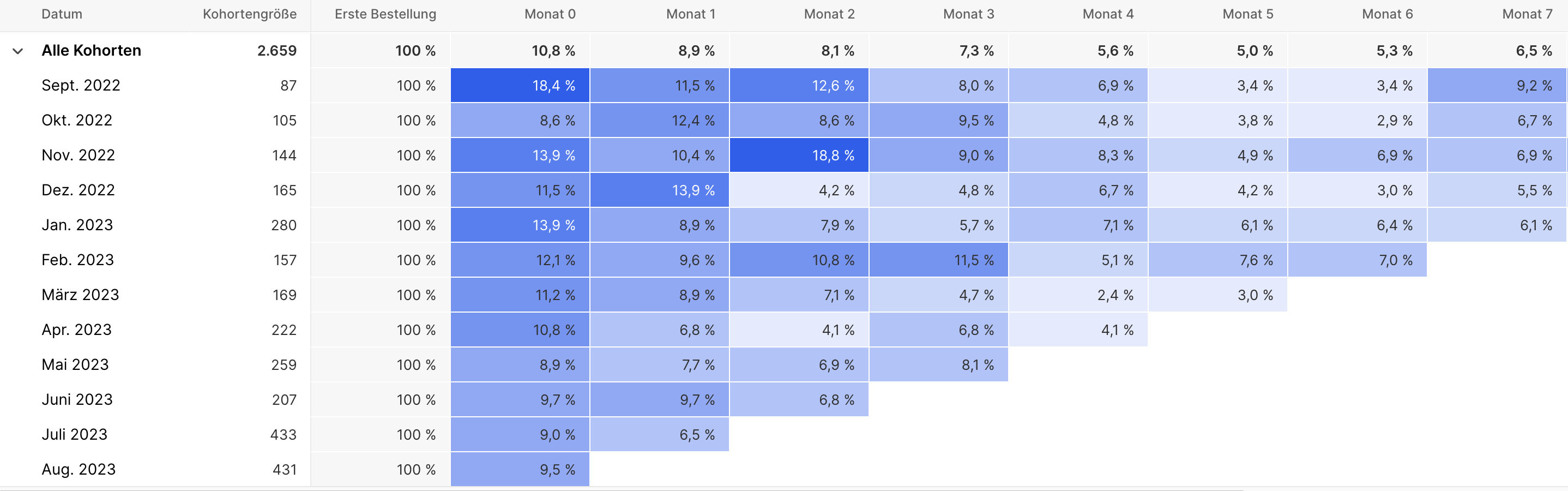
Unfortunately, the very rigid setting options do not allow detailed evaluation of customer behavior. This is where the use of RetentionX comes into play.
It's also important to keep in mind that interpreting cohort data is an iterative process. As you gain more insights and take action by adapting your marketing strategy, it's important to continue to track and analyze customer behavior to ensure that the actions you're taking are having the desired effect.
Here are a few key steps to take when interpreting cohort data:
- Identify key metrics: Identify the metrics that are important for your business, such as customer retention rate, purchase frequency, and purchase value. These metrics can provide important insights into customer behavior and should be analyzed for each cohort.
- Look for patterns and trends: Analyze the data to identify patterns and trends that can inform business decisions. For example, you might notice that a particular customer cohort has a higher lifetime value than others, or that a different cohort is at a higher risk of churn.
- Compare cohorts: Compare the different cohorts to identify similarities and differences in customer behavior. This can help you understand which cohorts are most valuable to your business and which are at the highest risk of churning.
- Look for outliers: Identify any customers or groups of customers that have particularly high or low values for key metrics. These outliers can provide valuable insights into customer behavior and can help you identify potential areas for improvement.
- Identify cause and effect: Identify any potential cause and effect relationships between different metrics. For example, if you notice that customers who have a high purchase frequency also have a high lifetime value, you can assume that the high purchase frequency is the cause of the high lifetime value.
In conclusion, while Shopify's built-in cohort analysis capabilities serve as a starting point for businesses, the platform's limitations become apparent when compared to the deeper, more nuanced analysis offered by RetentionX.
-
RetentionXMaximize Your Lifetime Value (LTV) with Analytics & Automation
30-day free trial
Free plan available
Built for Shopify Plus
Shopify Cohort Analysis is an effective tool for grouping customers and tracking their behavior over time.It helps businesses identify valuable customers, optimize marketing campaigns, personalize customer experience, and gain insights to inform business decisions.Alternatives such as RetentionX & Peel Insights offer enhanced cohort analysis capabilities with advanced metrics to maximize customer engagement & increase revenue.
What is cohort analysis in Shopify?
Cohort Analysis in Shopify is a chart that tracks how customer cohorts progress based on their first purchases and repeat purchases data. Each row reflects the customers of one cohort month-by-month, with each column showing pertinent information about these groups’ purchasing habits.
What is an example of a cohort analysis report?
Cohort analysis is a method to observe how customer behavior fluctuates over time, such as their rate of ordering or the average value per order. An example would be if a shop sorts customers into categories based on when they first purchased from them and follows up by monitoring each cohort’s activity throughout the months following that initial purchase.
How do you create a cohort analysis?
To create a cohort analysis, begin by taking in sales or transactional data and adding a specific column for customers to be grouped into cohorts. Create the necessary PivotTable alongside one devoted to retention rates - along with identifying multiple cohorts over time via the ‘Months’ sheet, in order to then calculate customer retentions each month as well as their respective percentages.
What is a customer cohort analysis on Shopify?
Customer cohort analysis on Shopify is an effective tool to observe customer retention and buying habits. Through the analysis, loyal customers are sorted based on when they made their first purchase. Data about repeat purchases can then be seen through a cohort table. Metrics like net sales, gross sales and average order value will also be visible in this report for Review of customers’ behaviours over time. This way we gain valuable insights into our customer base from examining purchasing activity by individual cohorts such as those that have made their initial purchase at different points in time or with certain attributes attached to them.
How can cohort analysis help identify valuable customers?
Cohort analysis is a powerful strategy for analyzing customer value by examining their recurrent buying activity and choices. This can help identify which customers are more inclined to make Purchases in the future, providing invaluable insight into how best meet their needs via cohort segmentation.